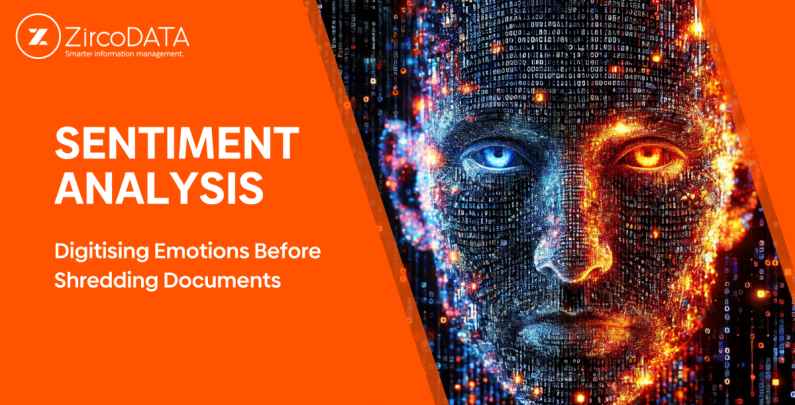
Understanding Document Sentiment Analysis and Its Growing Importance
Every document tells a story—but are businesses truly listening? With sentiment analysis, businesses are discovering new ways to listen more closely than ever before.
In today’s era of Big Data, organisations are moving beyond simply collecting information. They’re beginning to uncover deeper meaning and emotional context within the vast volume of text-based records generated daily. From customer feedback and legal contracts to compliance reports, written content holds invaluable insights—yet traditional analytics often overlook the human sentiment woven throughout.
That’s where document sentiment analysis comes in. Using AI-powered Natural Language Processing (NLP), businesses can decode emotional tone, context, and intent from their records. Rather than viewing documents as static data, they can extract actionable intelligence to improve customer experiences, enhance compliance strategies, and support more informed decision-making.
As organisations deal with information overload, having structured, well-managed records ensures these critical insights aren’t lost before documents are digitised or securely disposed of. The ability to analyse sentiment at scale has become a competitive advantage, turning raw text into a strategic business asset—raising a timely question: is technology finally bridging the gap between data-driven analysis and human emotion?
How Document Sentiment Analysis Works: Methodologies & Tools
Understanding the mechanics behind sentiment analysis reveals how complex emotions in text can become actionable data. Unlike basic word recognition, which lacks nuance, modern sentiment analysis blends machine learning, NLP, and contextual understanding to identify whether the sentiment of a document is positive, negative, or neutral.
Key Components of Sentiment Analysis
- Text Preprocessing: Before sentiment analysis can begin, documents must be cleaned and structured. This includes removing special characters, correcting grammatical errors, eliminating stop words, and ensuring the text is formatted for optimal machine readability.
- Tokenisation & Feature Extraction: Breaking down text into individual words, phrases, or key elements allows algorithms to recognise patterns and associations. Feature extraction helps identify sentiment-laden words and their frequency, enabling a more accurate classification of the overall document tone.
- Machine Learning Models: AI-driven sentiment analysis relies on trained models that classify sentiment based on historical data. This includes supervised learning, where models are trained on labeled sentiment data, and unsupervised learning, which detects patterns and clusters sentiment trends without predefined labels.
- Contextual Understanding: One of the biggest challenges in sentiment analysis is avoiding misinterpretation. Words can have different meanings based on context, industry jargon, or tone. Advanced AI models incorporate Natural Language Processing (NLP) techniques to recognise sarcasm, detect nuanced sentiment, and ensure more accurate results.
- Aspect-Based Sentiment Analysis (ABSA): Sentiment isn’t always applied to an entire document as it can be tied to specific aspects within it. ABSA helps businesses understand sentiment related to particular products, services, or business functions through sentiment segmentation, rather than just generalising the entire text’s emotional tone.
- Real-Time Sentiment Monitoring: Businesses increasingly rely on real-time sentiment tracking to monitor customer reviews, social media mentions, and market trends. Modern document sentiment analysis tools use AI-powered dashboards that provide instant insights, helping organisations make preemptive and agile decisions based on emerging sentiment trends.
Some of the most commonly used sentiment analysis documentation tools include Google’s Natural Language API, IBM Watson Tone Analyzer, and Microsoft Azure Text Analytics, each offering advanced features tailored for various industries. These tools are revolutionising the way businesses interpret written content, turning everyday documents into powerful sources of insight that drive smarter strategies and innovation.
Real-World Applications: How Businesses Leverage Document Sentiment Analysis
Enhancing Information & Records Management
For businesses handling large volumes of confidential records, ensuring compliance, security, and efficiency is paramount. Document sentiment analysis helps organisations assess the sentiment and tone in client correspondences, contract negotiations, and compliance reports, allowing them to streamline document workflows, enhance risk detection, and ensure regulatory adherence. Integrating sentiment analysis with robust records and information management solutions ensures that organisations maintain structured, secure, and accessible documentation while extracting actionable insights from critical data.
Risk Mitigation in Banking, Insurance & Corporate Governance
Industries dealing with financial transactions, regulatory compliance, and fraud prevention depend on textual documentation for risk assessment and fraud detection. Document sentiment analysis enables banks, insurance firms, and corporate compliance teams to extract critical insights from financial reports, claims documents, audit records, and regulatory filings. This helps flag high-risk transactions, detect anomalies in compliance records, and identify fraudulent activities before they escalate, strengthening overall risk management frameworks.
Legal & Compliance Risk Management
In legal and corporate compliance, sentiment analysis plays a crucial role in contract risk assessments, dispute resolution, and compliance monitoring. Document sentiment analysis helps law firms and compliance teams detect early warning signs in employment disputes, contractual risks, and policy violations, ensuring proactive risk mitigation. A real-world example comes from a study on Canadian maritime case law, where AI-driven sentiment analysis using deep learning models uncovered hidden biases and sentiment trends with 98.05% accuracy. By leveraging these technologies, legal teams can analyse vast amounts of case data, enhance decision-making, strengthen regulatory compliance, and proactively mitigate risks.
Improving Patient Care in Healthcare & Pharmaceuticals
The healthcare sector generates vast amounts of patient feedback, regulatory reports, and clinical trial data. Document sentiment analysis helps healthcare providers and pharmaceutical companies extract key insights from patient reviews, doctor feedback, regulatory filings, and medical case studies. This technology aids in identifying concerns about treatment effectiveness, detecting emerging healthcare trends, and improving overall patient care. For pharmaceutical companies, sentiment analysis is also crucial for analysing clinical trial documentation and ensuring compliance with evolving medical regulations.
Enhancing Security & Confidentiality in Information Governance
For organisations managing sensitive data and classified records, integrating document sentiment analysis into their information governance strategies is essential for enhancing security and compliance. By analysing security reports, data breach logs, and compliance audits, this approach helps uncover potential insider threats or risks. AI-driven Network Detection and Response (NDR) solutions are an example of how organisations are leveraging machine learning to monitor network traffic, detect anomalies, and identify potential security risks in real time. These advanced systems provide continuous threat detection, rapid incident response, and enhanced visibility into network activities, strengthening overall data protection strategies.
Challenges and Limitations of Document Sentiment Analysis
While document sentiment analysis provides powerful insights, interpreting human emotions through text remains a highly complex challenge. Language is inherently nuanced, influenced by tone, intent, and external context, making it difficult for AI-driven models to consistently capture sentiment with precision. The interaction between NLP, machine learning, and linguistic variability introduces layers of intricacy that require continuous refinement.
- Language Nuances & Context: Sarcasm, idioms, and cultural expressions can mislead AI algorithms, leading to misinterpretations and inaccurate sentiment classifications. Even humans, despite developing linguistic shortcuts, contextual cues, and emojis to convey emotions in text, often struggle to interpret sentiment accurately.
- Industry-Specific Terminology: Sentiment scores can be skewed or misclassified when AI lacks an understanding of domain-specific language, technical jargon, or industry slang.
- Data Bias: Sentiment analysis models rely on historical datasets for training, but if those datasets contain biases, the AI will mirror and amplify them.
Leveraging Document Sentiment Analysis for Smarter Decision-Making
Document sentiment analysis is reshaping how organisations interpret text, helping them spot trends, assess risks, and strengthen compliance. However, its effectiveness depends on the quality, organisation, and security of the documents being analysed. Even the most advanced AI tools struggle to generate accurate insights without structured, well-managed information.
ZircoDATA’s secure, compliant, and efficient records management solutions help organisations take control of their critical documents, optimising them for analysis, security, and long-term accessibility.
Recent Comments